This post originally appeared on MassBigData.org on August 15, 2016 as part of the Massive Data Blog Series.
On July 12, more than 120 data scientists converged on Pixability HQ in Boston for “Building and Managing Data Science the Right Way,” a meetup that featured a panel of data science leaders who discussed how to maximize data science’s impact on an organization. The packed house reflects just how much the data science community is flourishing in the greater Boston area. The panel discussed key challenges facing data science teams today, and shared expertise to help both new data scientists and veterans of the industry.
What makes a successful data scientist?
Panelists agreed that data scientists need to have quantitative ability for modeling and experimentation with data, as well as hacking ability to automate analysis with scripting languages like Python or R – see Figure 1. There’s no shortcut to developing a holistic quantitative skillset — it comes through building and modeling data sets over a long period of time. Data science is defined by more exploratory work compared to business intelligence, which by contrast is typically used to explain past data or insights. An understanding of programming and mathematics is essential for those embarking on their career: it’s crucial to develop hacker skills, get a degree in data science if possible, and study computer science to cultivate the skillset needed to manipulate data from a variety of different sources as well as select the right model for predicting or optimizing.
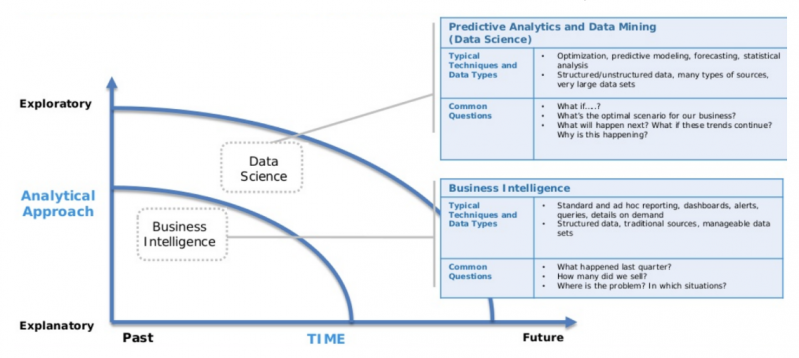
What makes a successful chief data scientist?
A Chief Data Scientist must, first and foremost, understand how the tactical and strategic applications of data science impact the bottom line. They must constantly be on the lookout for new sources and ways of synthesizing information to support other departments. These leaders need a business background and a hacker mindset — they should have the quantitative knowledge to suggest the appropriate analysis or modeling method, but also understand how a given approach can influence new products or features. For example, early on in my career, I was faced with the difficult problem of configuring routers and directing internet traffic around the world. I found that by using data science and automation, I could predict and route traffic based on past usage. It’s not just about the model — it’s about having a holistic knowledge of the model’s application, as well as the benefits, features, and advantages a model will produce.
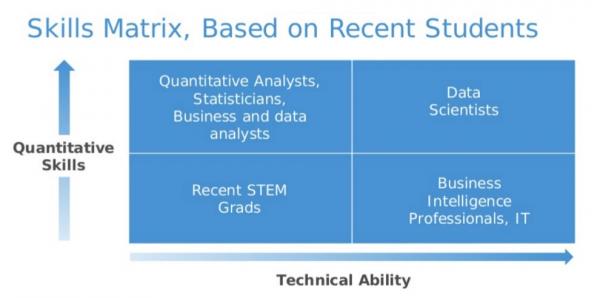
Where should the team sit?
While everyone on the panel was in agreement about what makes a data scientist great, there was little consensus on where the data science team belongs within an organization. Some voiced that the team belongs within engineering, while others stressed it should serve as its own department to encourage more rapid experimentation. While Pixability’s data science team is housed within the technology department, it functions as its own research and development team. This structure gives the team the autonomy to produce a significant amount of Pixability’s intellectual property. Within this structure, the data science team gives Pixability a leg up over the competition, helping clients make smarter ad buys, and supporting analytical requests from other departments, while also building groundbreaking new technologies like our cross-platform audience targeting engine.
How should the team choose tools?
Across the board, the panelists expressed similar challenges: saving large volumes of data in various formats; quickly creating visualizations; and needing to create, manage, and evolve code or models. But different teams require different tools to solve these problems. While one team has a massive amount of historical data to process, another team needs to acquire and process data more rapidly, and another team doesn’t even use databases because their data is constantly changing in real-time. At Pixability, we use Python for modeling data, Vertica as a database, Kafka to move data around, Spark to process it, Jenkins for data sourcing jobs, and Tableau for visualizing data. These powerful tools allow Pixability to manage years of data on audience groups and targeting across premium video platforms, while adding to the data set every day.
It’s an exciting time for data science — technological advances have led to an explosion of information worldwide, and with an immense amount of data generated daily, every tech company is now competing on its ability to manage and process this data. High-performing data science teams create a major competitive advantage for companies by automating data manipulation. Through strategic management of teams, tools, and information, data science leaders can empower their companies with analysis, models and systems to help outperform the competition.
To learn more about the impact of Pixability’s data science team, read about our new ground-breaking audience targeting solution.